What does it mean to be an AI hub for Singapore?
With AI being a buzz word these days, there been much discussions lately into turning Singapore into an AI hub. But what do we mean by that?
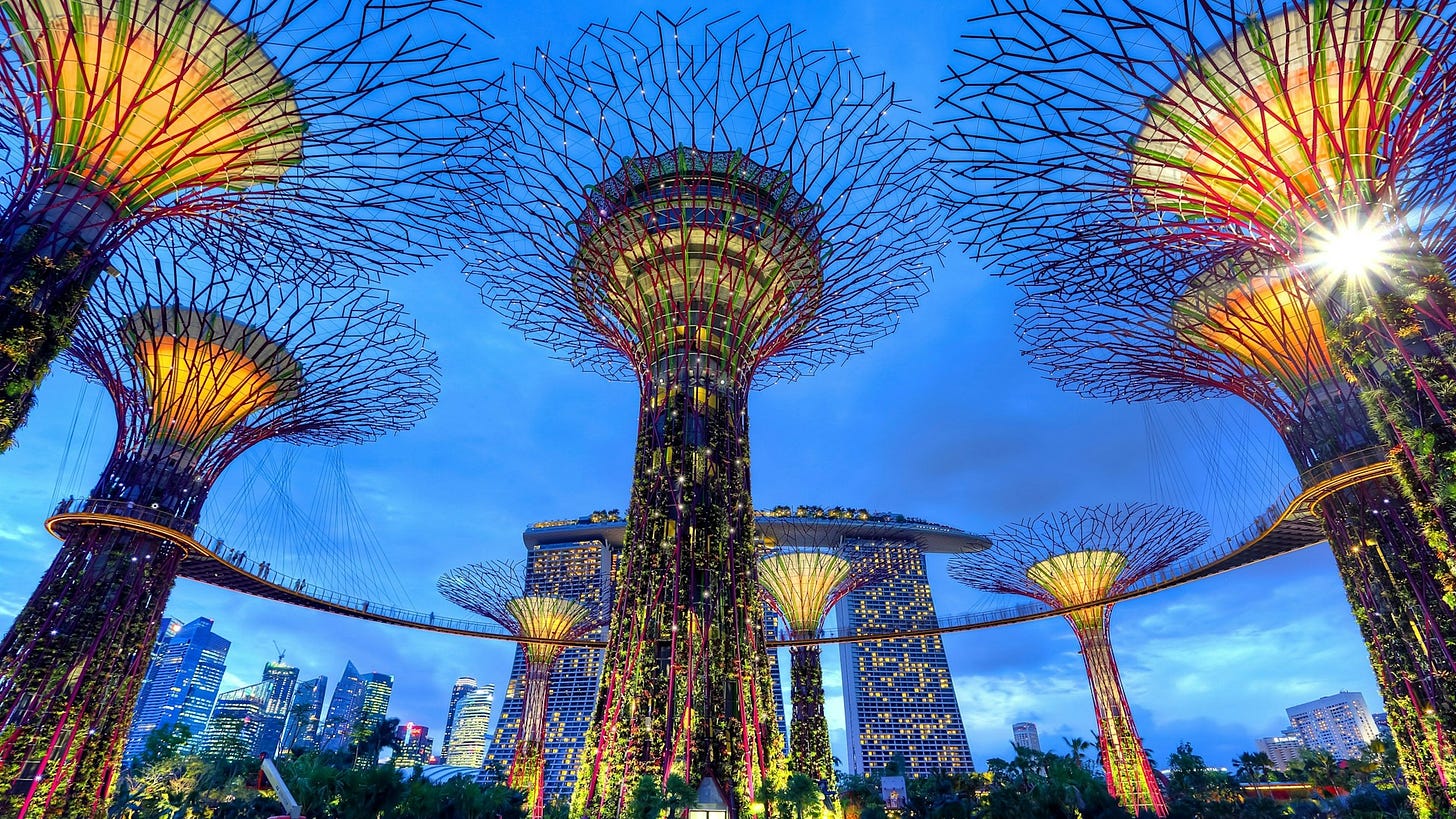
🇸🇬🇸🇬🇸🇬 National specific content 🇸🇬🇸🇬🇸🇬
The following article was specifically written for Singapore audience where I am born from. And may or may not be applicable to my wider audience (hi to the rest of the world)
Additionally, because this is targeting a Singapore audience, we will be using the local terminology of AI Creator (Foundation model makers), and AI Practitioners (More commonly known as AI Engineers)
It also comes in two parts
- Part 1: Explores more on what it means to be an AI hub, and is meant to be a primer for a more general audience, before part 2. (this article)
- Part 2: Goes in depth, into a blueprint for turning Singapore into an AI hub
With AI being a buzzword, much lately has been discussed about turning Singapore into an AI hub.
With the national AI strategy goal of building over 15,000 AI creators and practitioners. We must be clear about what we mean when we say AI hub.
To do so, we shall explore the various hubs that exist today. In the AI world.
But before that….
Let me correct 3 common AI scaling misconceptions…
Because AI is a completely new field to most people, it would help if I outline a few major things, that are commonly misunderstood. And it is typically all about scales.
Common misconceptions that commonly baffle, everyone else both at the highest level of government, to individuals outside the AI field.
And it’s all about scales …
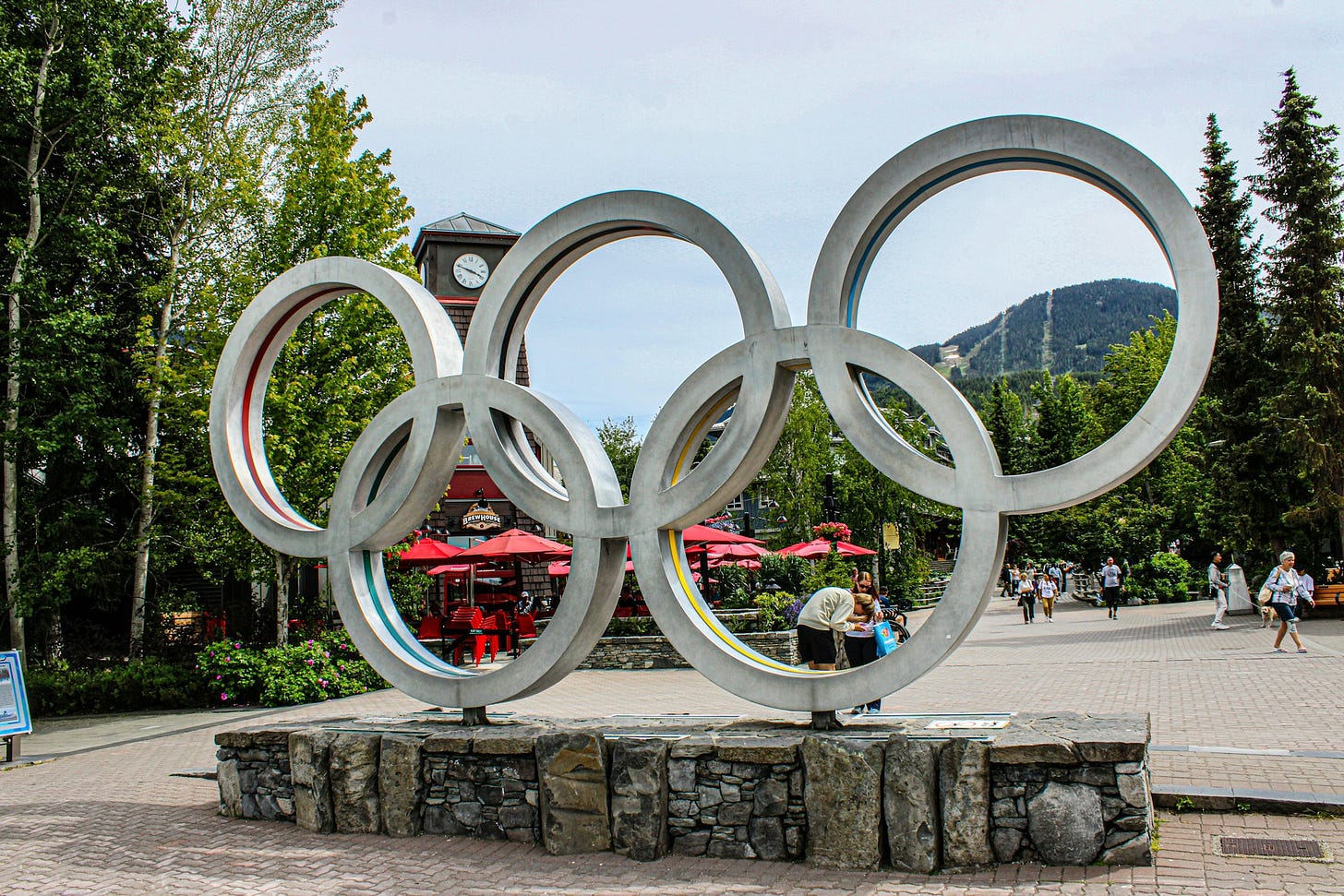
1) AI Creators are rarer than Olympian Athletes.
There are approximately less than 50 AI engineering leaders out there today - who can make a new large language model from scratch.
With less than 1,000 Individuals worldwide who can contribute to such teams. Globally.
So to start it off - if the plan was to have 15,000 AI creators in Singapore, that is unrealistic at best, because that would mean bringing the entire AI creator global talent pool into this island. (it definitely can be an aspiration if we choose to do so)
Thankfully, it seems like the people behind the National AI Strategy are at least aware of this constraint.
However, I bring this up - as this rarity, is a blindspot for, any investors, or policymakers. Because it can be too convenient to think in terms of “Just get some engineers, data, and GPUs”.
To build an AI model that is larger than 7 Billion Parameters today correctly, across more than a Trillion tokens (Which I would use as a loosely defined bar for a “Barely usable foundation model”). Without burning away a million dollars or more in waste.
Would require skillsets from various domains, from dataset to model architecture, to training infrastructure. And due to the scarcity of talent in these domains, and a further scarcity of individuals with cross-domain knowledge and team leadership experience.
Who can lead a team, and create a foundation model project from start to finish?
Overall, we are talking about: 0.00000633% of the world population for AI engineering leaders. Fewer AI creators, than the number of athletes competing in the Olympics today.
In that lens, it is arguably easier to win a 4D lottery, than to find and recruit such individuals, to build and lead teams locally in Singapore.
The reason for this scarcity comes from two major reasons
The high cost of doing experiments at such sizes = High cost to learn
Lots of design and parameter choices, from dataset to model. The lack of scientific historical data, today means a decision has to be made in equal part “artistic decisions” and “scientifically data-driven informed decisions”
As a result, very few individuals afford the benefit of learning from experience (and/or mistakes), in leading such a project or be a part of existing training projects. Especially when such experiences sometimes require a 100k++ training bill.
What further this scarcity, is how the vast majority of them will be locked up in major tech companies, and/or China.
As such. When the opportunity arises to invest and anchor them to be champions in Singapore. To build and grow AI practitioners around them - As part of our larger AI strategy. We should do so.
Notable example: Yann LeCun who leads the Facebook AI team, as one of Canada's Anchor and Champion to the pool of ~1,500 AI Creators. Alternatively, Mistral is the anchor for France.
Considering the rarity, it is easy for VC’s and Government officials to fall into the thinking of “there will be another opportunity or later”
Or defer the opportunity out of concerns regarding “unclear AI future”
And never get such an opportunity again for years.
The thing is, in a world where no one can be certain what will happen next in the field. The winners would be among those who play an active part in building this future, figuring out the answer along the way.
Before the rest of the world understand, or take action, when it is might be too late.
Like athletes, these are teams you invest in, to take a shot of leading the world stage. Without ever knowing the certainty of success of any one team, until the race is finished.
The champions, to inspire the rest of your industry / athletes
2) We do not need a multi-billion-dollar GPU cluster
It’s more a nice to have than a need.
Broadly speaking, AI models can be viewed across the following stages
Pre-Training
Alignment / Fine-tuning
Deployment
In only the later 2 stages, is it more desirable for it to be done “locally”, and broadly speaking we would need “just enough GPUs”.
Pre-Training
is the only phase that requires thousands of top-of-the-line GPUs, with dedicated power and networking, to be all centralized in a single location.
This phase is highly constraint by energy cost, and typically uses largely public data for the training process. As such, it is location-independent and can be performed remotely, anywhere on this earth.
This, as a result, biased foundation model training, towards cheaper low-cost energy or cooling.
Because Singapore is not naturally cooled like Greenland or Finland. Nor do we have a large quantity of cheap electrical energy. There is little to no reason why Pre-Training “must” be done in Singapore itself.
(eg. I use Finland servers significantly, but never been once to the country)
Alignment / Fine-tuning
The next phase is when Model practitioners, or AI Engineers, take existing models, trained on trillions of parameters. Fine-tune and align such models towards a specific goal or task. With a small cluster of GPUs across thousands to a few million tokens.
During this phase, it may be common for more sensitive/private data to be included. For example, a hospital fine-tuning a medical model may be using private datasets that are not allowed to leave the nation.
So, while there is still a need for high-end GPU, with costly GPU interlink at this phase, it is significantly smaller (and cheaper) than the pre-training phase.
Deployment
After all the training, fine-tuning, and alignment. The next step is deployment. For multiple reasons, be it the latency of the request, or the legal sensitivity of personal information being used in the request.
There is a desire to bring the deployment within the same nation.
Typically at this phase, we avoid costly interlinks unless strictly needed by the largest of models, and for smaller models, may even use smaller more cost-effective GPUs instead.
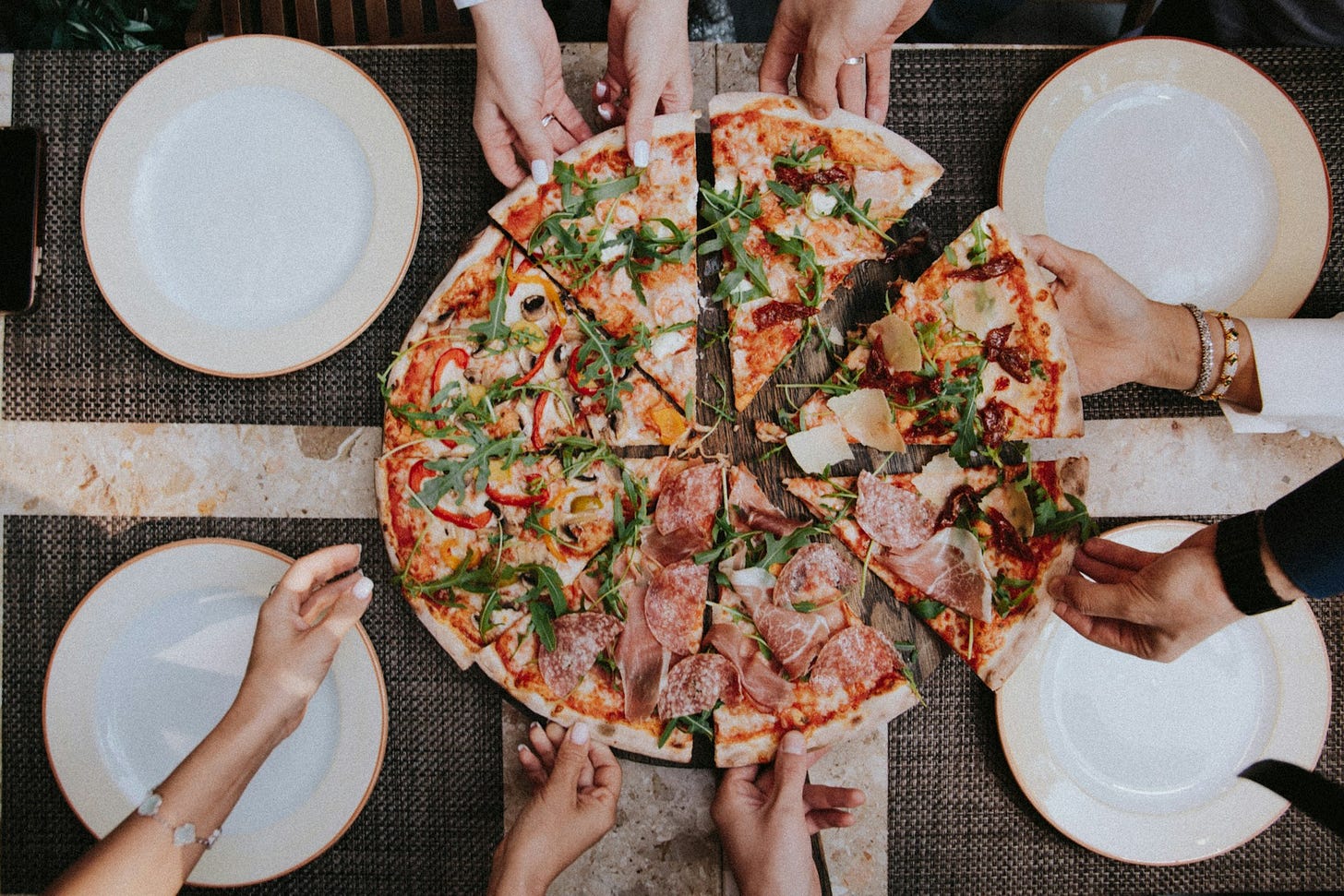
3) We do not need a large team to do XYZ
One of the big trends in AI is how AI project is defined more by individuals and smaller teams, than large organizational teams.
Because a large % of their budget is spent more on computing, GPU, and supporting resources. AI-first teams tend to be substantially smaller than your typical development team.
Notable examples of this are Reeka and DeepSeek, with less than 50 people. Created foundation models from scratch, comparable to GPT 4. Midjourney is less than 20, The foundation model team for RWKV (which I co-lead) is less than 10.
It matters more what the individual contributors do, test, and decide on for an AI project, in a way that can be scaled across multiple GPUs. Then a large organization of individuals collectively.
Outside of the AI model creation space, and into the practitioner space, we have started seeing the rise of AI Practitioners, who effectively create entire million-dollar companies, by themselves, or with teams smaller than 10.
Putting the above 3 scales into context
Realistically, to build a 15,000-strong pool of AI Creators and Practitioners. We are looking at most some combination of the following…
3-10 AI Creator teams, of 10-100 individuals (< 1,000 total)
30-100 AI Practitioner Tooling teams, of 5-100 individuals (< 5,000 total)
200-500 AI Practitioner teams, of 1-20 Individuals (~12,500 total)
Additionally, Our existing national GPU cluster plans are probably enough when fully utilized. We can subsequently let the private market fill in the gaps when needed.
With that out of the way, let’s define the type of AI hubs out there.
The 4 major types of hubs
With examples provided with each.
Canada: AI education and research hub
India / Taiwan / Guangzhou: AI Enterprise Solutions & Implementation outsource hub
Singapore Default role: A sales & management hub for South East Asia for big tech
San Francisco & France: A tech hub for new AI companies and startup
Strictly speaking, none of these options are mutually exclusive. But it does help guide the vision of what we would want to achieve as a nation.
Additionally, while we definitely can take a balanced bet on all 4 routes. If we want to succeed beyond our default role, we need to make sure we put in the required resources for other bets to succeed
so that we do not try to do everything, and achieve nothing.
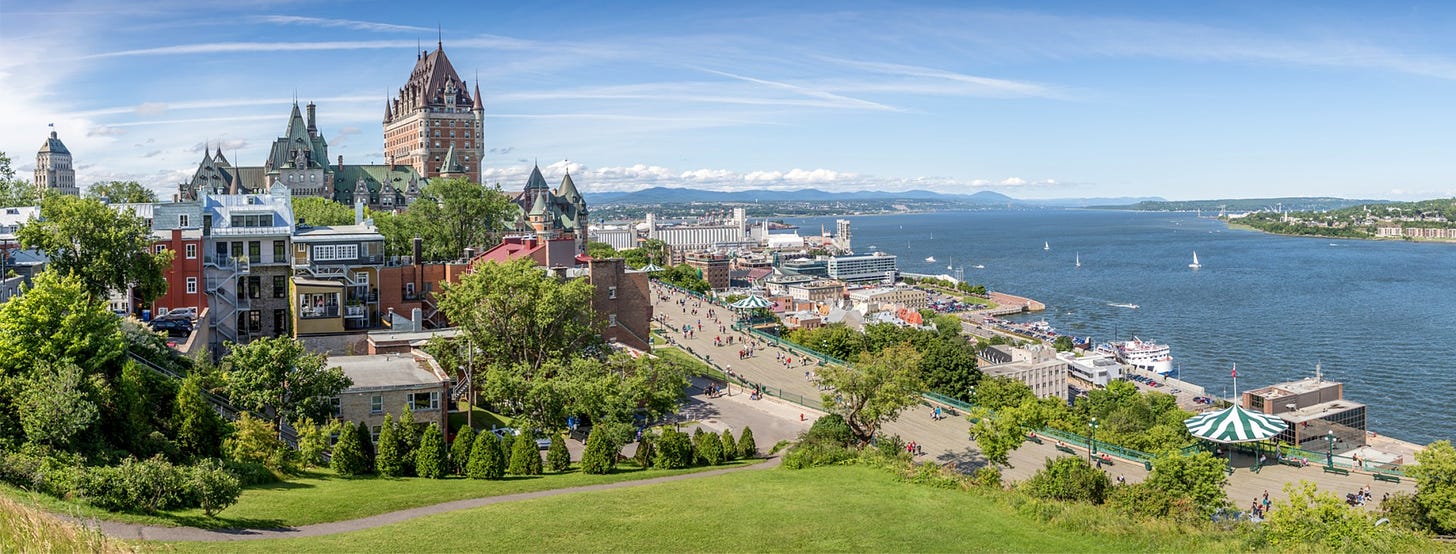
Canada: AI Education and Research Hub
Canada is currently well regarded in the AI space as one of the main AI research hubs.
All the major companies, like Google and Facebook, have set up their AI research lab with the universities. And pooled in close to $2 billion dollars, worth of investment into the area.
All so that they can have access to the growing pool of ~1,500 researchers and scientists, along with early access to the larger pool of 11,000 AI and data science students.
What is unique behind their success is how it was primarily about the concentration of talent.
The major companies were setting up operations, not because of the economic demand for AI in Canada, nor because of the startup ecosystem.
Just talent. Driven by prominent researchers at the top of their field, at Google, Facebook, etc. And proximity to the US timezone (and English!)
You can read more here on Canada investment and AI hub: https://www.investquebec.com/documents/int/publications/Brochure_IntelligenceArtificielle_web_AN.pdf
Challenges in replicating this hub today:
The era, where frontier research is in public, with universities.
Ended when open AI went closed source.
Pre ChatGPT, the bulk of AI research was done in universities, or jointly between commercial and public groups. It was common for the head of these research labs, to play an active role in the universities. Publishing papers jointly in the process.
Canada positioned itself as the ideal place for these researchers. By having one of the largest pools of AI students, which these companies potentially can recruit from. On the flip side, the students interested in AI research, came to the Canadian universities, as this was where the prominent head of research in multiple labs, was giving lessons from.
Post ChatGPT, various of the largest frontier AI groups, in particular the closed source ones, now avoid publishing papers, and avoid working with universities. Making it particularly hard to bootstrap this very same process from scratch.
But possibly doable, if we focus on collaboration with multiple hubs, as a means to bootstrap our education hub in AI.
Also, notice how it’s only ~1,500 researchers. And it’s considered one of the biggest hubs out there.
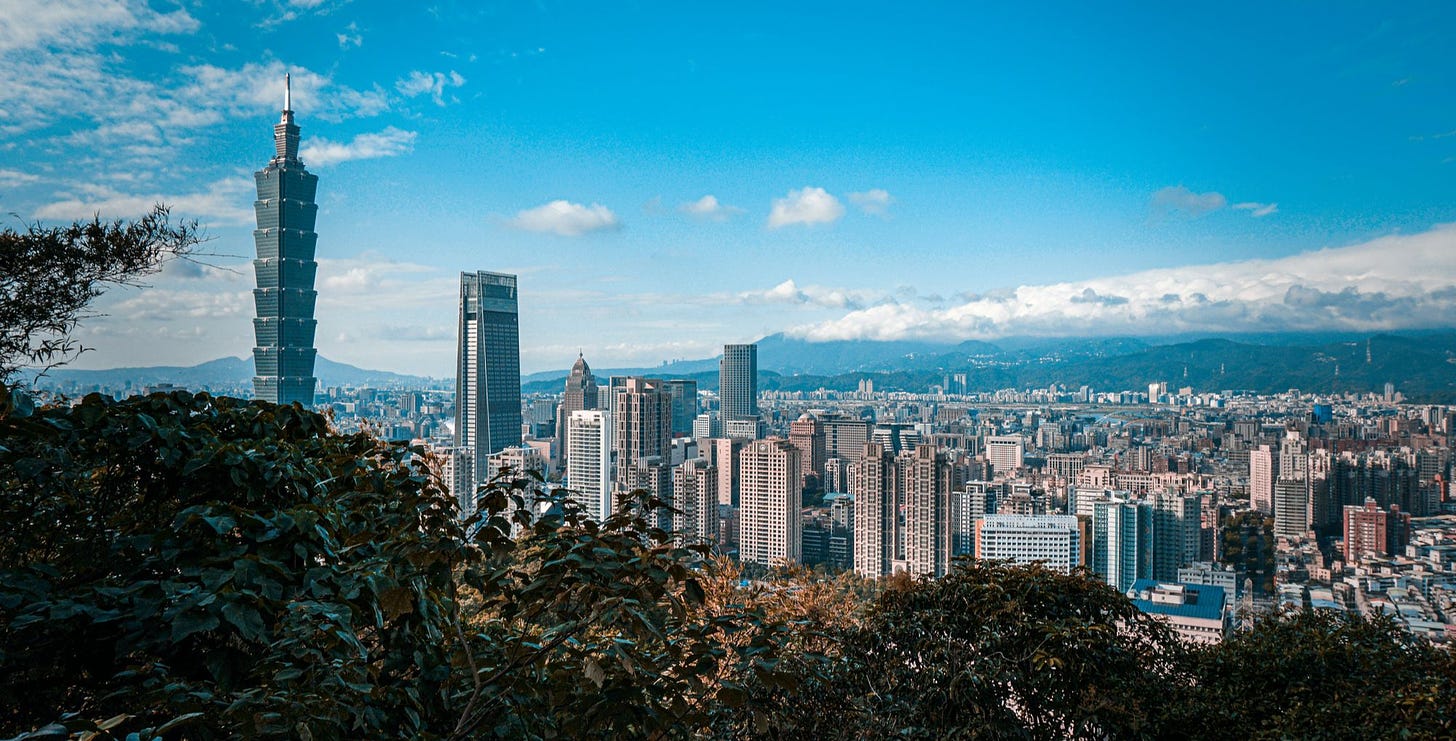
India / Taiwan / Guangzhou: AI Enterprise solution and implementation outsource hub
It’s no secret enterprise is spending lots of money on custom AI solutions. And are turning to their traditional solutions providers for answers in implementing their AI solutions.
To the tune of over $2.6 billion a year, for just Accenture alone. Who only represents a fraction of the overall landscape of hundreds of IT solution vendors.
So while India / Taiwan / Guangzhou, may have a limited pool of AI model creators. They have since rapidly up-skilled their pool of Software Engineers to AI engineers and practitioners, to serve the growing demand of enterprises for AI solutions.
For India in particular, this is bolstered by the recent domestic national focus on Sovereign AI solutions, due to their own national language barrier, and the desire to move away from both US and China-based solutions, due to geo-political reasons.
Challenges in replicating this hub today:
The bigger the company headcount is
the more it’s just a number
the more it’s just about the $ per person
This is the culture of large enterprises, which unless we can change even within our government and large institutes, we are doomed to repeat again.
Collectively, India / Taiwan / Guangzhou currently holds their position as the world’s Enterprise Solution workforce hub, due to their large pool of technical workforce, at affordable prices.
The current demand for their AI practitioners has been an organic shift from Software Engineers, as demanded by their solutions providers.
Besides India, another growing segment lately has been Taiwan / Guangzhou. Multiple enterprises within Singapore outsourcing their development workforce to Taiwan / Guangzhou.
All of this is largely driven, by how enterprises tend’s to generalize headcount as interchangeable. As such salary price has been the number one driver of this movement - which will not be working to our advantage.
I do not have a clear answer to this problem. Beyond “somehow, magically” changing large organization mindsets.
And this is being said as someone who has worked as a vendor to 90+% of all the banks and financial institutes in Singapore and has been part of the enterprise system.
The cultural thinking of developers and engineers being equivalent or a cost center in the organization - instead of talent and asset - is a complex problem in itself that runs on a global scale.
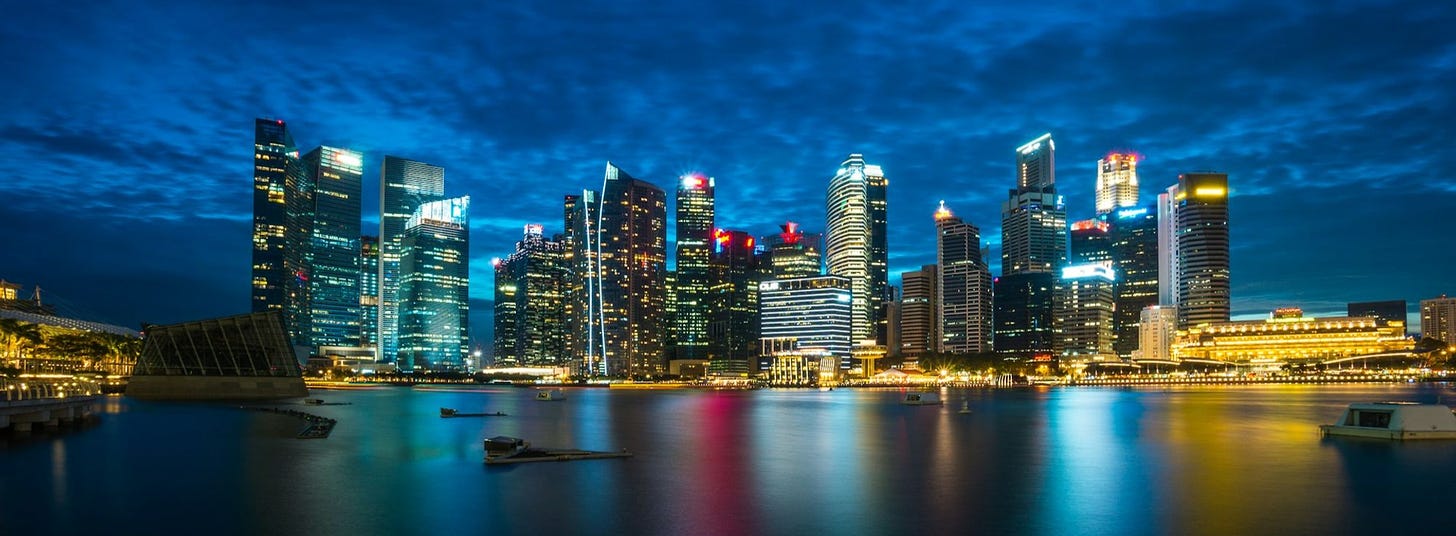
Singapore Default Role: Sales & Management hub for South East Asia for big tech
Because of India, Taiwan, Guangzhou, and economic salary advantages. They ended up as the outsourced development hub.
Instead of competing head-on, what ended up in the software development scenes, was more enterprises leveraging Singapore as a Management hub to bridge the gaps between the software developers overseas to their customers in the region.
So while there are still developer talents hired locally, they are pushed to either manage their overseas team, or work on more sensitive, or contextually local work. Leaving the bulk of the work to their overseas outsourced development hub.
And perhaps this is alright? If this is our goal.
It’s what helps entrench our positioning as the hub for South East Asia and helps strengthen our finance sector, as all the capital flow for these operations would be flowing in and out of Singapore.
Challenges in replicating this hub today:
There is not much to be added, this is the default for us, as long as we do not be “complacent” on it. And continue our investments down this path.
Due to our strong financial services, and legal framework. This has already been our default role for software development for big tech in the Asia region.
However, this route is not going to help us create a hub of 15,000 AI Practitioners and Creators. It will more likely instead create a hub of 1,000 AI Project Managers - 2,500 AI Practitioners locally - and 15,000 Practitioners overseas.
While it will help continue to grow our finance services, most of the value and benefits to the economy will be routed overseas. While substantial, it will not be the new “Engine of Growth” we need.
But I would ask? Do we want to let the default happen?
Is this what we desire to be as a nation?
Where we are simply followers who are at the mercy of the major AI companies
Or do we want to take the lead and control our future, in AI?
One is a low-risk, low-effort, safe bet, that will give us modest economic benefits, which will certainly happen. The alternatives are huge expensive bets, that may be a new engine of growth if done right. But a waste of capital if failed.
Luckily for us, even if we make big bets, this default will still happen. Or may even be bolstered.
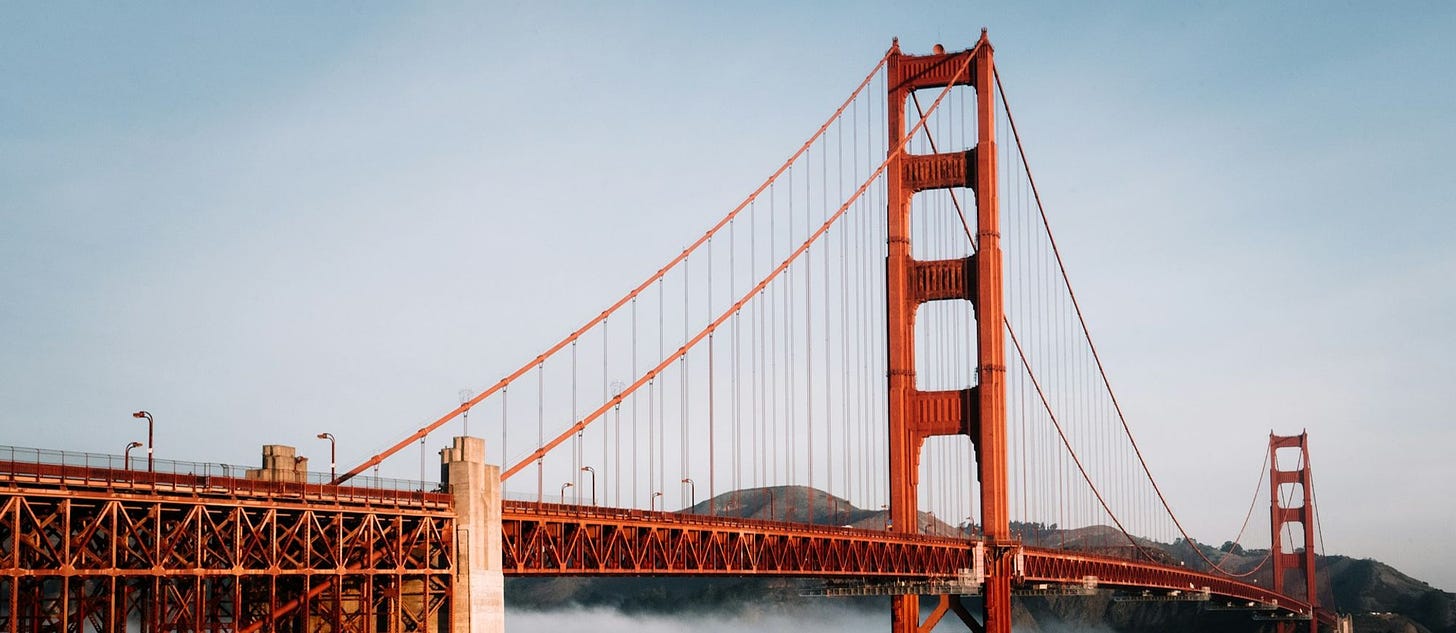
San Francisco & France: A tech hub for new AI companies & startup
We have seen the rapid multi-billion dollar investments and the rise of multiple startups in both France and San Francisco. Both big and small.
Every nation wants to have such a hub that has been built. In their local country and economy.
As it brings in the investors, talent, and the people.
And it is, at a mind-boggling scale. San Francisco companies receive 50+% of all global funding in AI, or US$ 27 Billion+, with France and China following behind.
And despite San Francisco sky sky-high salary costs, the reason why talent is retained and attracted is due to the ecosystem that has been built.
The reason why these startups and new AI tech companies do not overly outsource like Enterprises is because the individual contributor matters more than the head count. Where the individual salary cost matters less than the GPU costs.
I will be covering more about San Francisco and France in a follow-up article. So as such, I will leave it as it is. That it is currently the AI hub the world wants to be.
Challenges in replicating this hub today:
Capital. Every country wants a San Francisco or France AI hub.
But they do not want to put up the >$1 Billion dollar investment required to make it happen.
Secondary Obstacle - Market & Talent.
But here is the crazy thing:
Singapore literally have all the infinity stones to make this happen - if we can put it all together, and “Snap” it into place.
Deciding between an Education hub, or a startup tech hub
Because we will become the sales and management hub for South East Asia by default.
And the fact is, we will unlikely win the outsourcing salary competition for enterprises. Due to many external economic factors.
Because of that, our options are to go beyond, if we want to build that 15,000-strong workshop. Is to either be the Education Hub, the AI startup hub, or both.
So my fundamental question is….
What sort of AI hub does the Singapore Government want Singapore to be?
An Education hub? A startup hub? or an outsourcing hub?
If it’s an AI education hub:
I am not a professor at a university. I cannot advise how to build Singapore into an Education hub. So I will be skipping that. (Maybe ask the professors in Canada)
But if it is an AI Startup hub:
Because I am a Founder, an Entrepreneur, and an AI Researcher. I can help answer that question next.
Signed Eugene,
From San Francisco